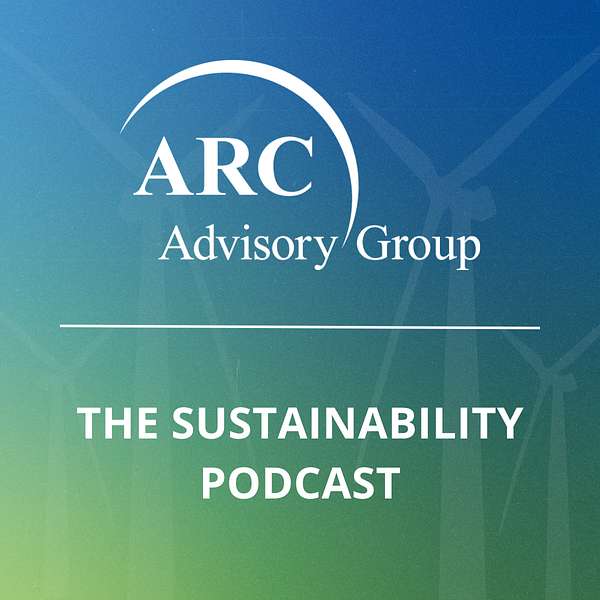
The Sustainability Podcast
The ARC Sustainability Podcast is an interview-based format where science, technology, and sustainability come together. Our conversations involve many sectors of sustainability such as supply chain management, ESG, the energy transition, industry infrastructure, and manufacturing. Through each discussion we reveal strategies, tools, and processes that leaders and companies are taking to achieving sustainability.
For More Information Visit us at: https://www.arcweb.com
The Sustainability Podcast
Industrial AI: Evolution or Revolution? Unveiling the Future of Technology in Our 5 Part Series
Welcome to a special crossover episode of The Sustainability Podcast, in collaboration with ARC's Digital Transformation Podcast. Join host Jim Frazer, Vice President of Smart Cities and Infrastructure at ARC, and Colin Masson, ARC's Director of Research and former Microsoft executive, as they dive into the world of Industrial AI.
This episode kicks off a five-part series exploring whether AI in the industrial sector is an evolution of existing technologies or a revolutionary leap forward. Jim and Colin discuss the history, tools, and techniques of AI, setting the stage for an engaging conversation on the future of industrial AI.
Don't miss this insightful discussion that bridges the gap between engineering rigor and transformative potential. Tune in for future episodes on AI wars, industrial use cases, data fabrics, and AI’s role in sustainability. Listen and subscribe on your favorite podcast platform, and watch the episode on YouTube.
--------------------------------------------------------------------------
Would you like to be a guest on our growing podcast?
If you have an intriguing, thought provoking topic you'd like to discuss on our podcast, please contact our host Jim Frazer or Our Producer Tom Cabot
View all the episodes here: https://thesustainabilitypodcast.buzzsprout.com
Jim Frazer (00:02)
Welcome to another episode of the Sustainability Podcast. In our AI series, we're actually very happy to be joining forces with ARC's Parallel Podcast, which is the Digital Transformation Podcast. If you haven't seen those or listened to those episodes, we urge you to also go to your favorite search engine and look for the Industrial Sustainability Podcast by ARC.
As many of you know, I'm Jim Frazer, Vice President of Smart Cities and Infra Structure here at ARC. And I am combining on both of those, on those two podcasts for a series of artificial intelligence. And our domain expert from ARC that will be joining me today and for the rest of these episodes is Colin Masson, Director of Research here at ARC. He's recently joined ARC from a long career at Microsoft.
Colin, welcome and how are you today?
COLIN MASSON (01:10)
I'm great Jim and I'm looking forward to this series. We've already done a couple of episodes on the Digital Transformation podcast channel where I really interviewed one of the first chief AI officers I've met and also CTO of one of our vendor clients. But I think here we're really going to set some strong foundations just to think about, and you called it AI, I think about it as industrial.
AI which is kind of going to be our launching topic.
Jim Frazer (01:45)
Correct, correct. Before we start diving down into the nuts and bolts of the technology, Colin, can you tell us a little bit about yourself and your background?
COLIN MASSON (01:48)
Before we start diving down.
Sure, like many of us at ARC, I have an engineering background, spend a lot of time...
chemical engineer by training, but I've spent a lot of my career across various verticals within the industrial sector, everything from consumer packaged goods, pharmaceutical, I've done a lot of work in discrete manufacturing, particularly in automotive and industrial equipment. But that career kind of spans practicing as an engineer to becoming a CTO, to becoming a CIO, and kind of actually returning to the analyst community where I spent some time before my last gig, as you called it, I think, which is really that last 15 years at Microsoft where I kind of worked very much on.
the emergence of cloud technology and kind of cloud and edge technologies, enterprise architecture, and of course, as we'll get into a lot on AI long before the emergence of generative AI.
Jim Frazer (03:09)
True, true. So in some of your writings during your brief term here at ARC, we've used the term, well, at ARC we've generally used the term AI, but there's an increasing focus about industrial AI. So can you describe industrial AI and how does it compare and contrast to, let's call it general AI?
COLIN MASSON (03:37)
Yes, we coined the term and kind of I used the term in my first strategy report for ARC, which is the Industrial AI Revolution. The R is in brackets. So we had a lot of internal discussion on.
how to frame AI with the emergence of, or the hype around generative AI. But what we really felt we needed to do was kind of remind everybody of two things. One, that really AI has been around for a long time and has been deployed.
quite broadly, not necessarily at scale, but lots of use cases of AI in the industrial sector. So we were to some extent trying to counter the hype around large language models and generative AI. The general popular press is kind of, doesn't always define what kind of AI or what specific tool or technique applies to solving a problem. So we kind of framed industrial AI.
and we've defined it in that first report, as really being a subset of all of the different AI tools and techniques, things that have been around for decades. I worked 25 years ago at Aspen Tech, right? And so we were doing multivariable control with neural net technology even back then, right? So neural nets, machine learning techniques, and how you train them with
supervised, unsupervised, reinforcement learning. All of those techniques have been around for a lot longer than, you know, 2022 in November when ChatGPT 3 .5 came out and suddenly people discovered generative AI, which in itself was really the transformer technology back in 2017. Google engineers kind of wrote the papers on applying those techniques. So,
We really wanted to have a term that just reset everybody and made them think about, all right, which AI tool or technique or which tool in the AI toolbox should you be using to solve a particular industrial use case?
And so that was really why we use the term. It's really to frame it in terms of there's more than generative AI, number one. And it's the set of AI tools that make the most sense for solving problems or achieving business outcomes in the industrial sector. And so not every tool will apply, but we...
Hopefully that answers your question, Jim. I could talk another 20 minutes on the whys, but that's the...
Jim Frazer (06:44)
That's a great start.
But that's a great start, Colin. Thank you for that. And I think you really led to my next question, which is you alluded and then explicitly talked about a set of AI tools, plural. So as a relative AI novice, I'm thinking, what are these? Is this various flavors of machine learning or of deep learning?
COLIN MASSON (07:06)
Mm -hmm.
Jim Frazer (07:17)
or data science and analytics or algorithms or just techniques. Can you talk about how, what is an AI tool? How do you define an AI tool?
COLIN MASSON (07:18)
data science and analytics.
We talked about the...
is an AI tool. How do you?
Yeah, I know I kind of popped up a slide behind me. Hopefully you're seeing that. And I know a lot of people will be listening to this podcast, so they won't be able to see it. But it's a good visual reminder to me of kind of the whole collection of tools and techniques that can apply to industrial use cases.
The way we kind of frame the impact of AI in the industrial sector is really by thinking about adoption of AI tools and techniques at scale. So I'll jump into those, which was really your specific question. But really thinking about their impact on people.
processes and that could be yes all the way down to the factory or energy production, oil and gas production and distribution.
but it could be the full set of business processes that any industrial organization has to deal with. So there's still the sales and service aspects of that. So people, processes, and then the other technologies, AI of course is one set of technologies, but really how they are going to impact and co -exist or augment other technologies over time. And so we kind of looked at a whole
set of tools and techniques. And so for those of you that can see the slide, I've kind of framed it around this core idea of an industrial data fabric. And again, we'll have a future session where we'll dive deep into what do we mean by that. But essentially, you've got foundation models that can be tuned and fine tuned using various techniques, RAG, which is kind of all the rage right now.
But it may not be large language models, it may be mixed mode models, it may be commercially available narrow models for time series data. For example, there are libraries of time series data models and some companies that are working specifically on that. So I've kind of got around that industrial data fabric, I've got this idea of all the open source and commercially available foundation models, large language models, industrial data sets.
that you may be able to get your hands on to train your AI models. And then I've kind of got all the different training techniques around that where we really talk about the ways that you can do unsupervised learning, deep learning, reinforcement learning, which is kind of one of the key techniques that has made OpenAI's CHAT GPT models is kind of one of the secret parts.
of the secret sources made them so successful and supervised learning and I mentioned unsupervised learning which kind of one set kind of uses labeling and actually there's quite a lot of hidden costs.
of people actually having to sit and look at mountains of data. This has been one of the things that's held back. Automotive and autonomous deep learning is the need to go and label lots of data, label a traffic sign or an unexpected object crossing the road, labeling those to train the models versus unsupervised learning which can kind of identify patterns and
and kind of learn without a lot of labelled data. So there's all those techniques, but then what are those techniques used to do? They're typically used to either deliver...
Jim Frazer (11:29)
true.
COLIN MASSON (11:38)
some form of language understanding or some form of object identification. So that's really where the terms natural language processing and computer or sometimes machine vision. So you'll see those terms used quite a lot and mixed mode where you kind of might be doing a combination of language, identifying an image or an object or even audio. Right. And so all of those
sensory inputs are really being identified and...
used to build specific skills using AI techniques. And then I've kind of got around that, by far not a complete list, but just I've got generative AI in that list of one of the things that people identify that typically is using large language models or.
building off of foundation models and some mostly large language, but some increasingly with mixed mode capabilities. But then there's other techniques that I think we're going to see a lot more of. So while the hype is around generative AI, you'll hear a lot of discussion around causal AI solutions and explainable AI solutions, which identify one of the key weaknesses in generative AI technologies built on transfer.
former technologies, which is really that we don't quite understand how they work. And so we can't say why did the AI make that recommendation or come up with that answer. And that's really what's behind some of these other techniques or other forms of AI, which really where you can get a root cause analysis or really trace back how was that recommendation from that core.
Jim Frazer (13:29)
good.
COLIN MASSON (13:41)
AI or explainable AI solution arrived at. So there's already a fair amount of causal AI solutions and vendors and researchers working on those technologies. But I think we're going to see a lot more of that partly because of the EU in particular has been...
quite quick to identify the potential problem in generative AI frontier or foundation models, these very large models that are being widely adopted in that you can't really understand why they made a recommendation. So they really have been pushing for this idea of explainable AI. So I think we'll see more and more, whether it's hardcore legislation or really just political
pressure, lobbyists, depending on how you want to think about it, pressuring us to really have more explainable AI solutions out there. Neuro -symbolic AI is another one that's very relevant to us in the industrial sector where really you use a combination of techniques that is better at math because I think we all know that
Large language models are really good at that, right? They understand and can interpret language and get quite creative about even helping us with language, but they're not good inherently at mathematics. And as engineers and working on mission critical processes, we obviously need solutions very often that have to be good at math. And certainly we don't want them hallucinating and making up
when it comes to mission critical engineering problems. So, neuro -symbolic AI is another one. And I put quantum AI on there as another one which will open up a whole class of, a new class of problems just as quantum technology itself will. So, I've kind of got all those things wrapped in that AI bubble that's intersecting with its impact on people, processes,
and technology more broadly. And if you think about the intersection kind of of those four bubbles, then at the center you've got that idea of an industrial grade data fabric which has to, can still use a lot of these AI techniques. In fact, we are extremely rich in data.
Data is not typically our problem. Maybe high quality data is very much a problem, but we have massive amounts of data that can be used to feed AI systems and be used to help train them on particular classes of problem.
Jim Frazer (16:45)
Exactly. Colin, I'm impressed that while we didn't even introduce the title of our first episode, it is in fact industrial AI, evolution or revolution. And it's clear from everything you've mentioned that AI is built upon a legacy of many decades of engineering.
COLIN MASSON (17:00)
Right. And it's clear.
of many decades of engineering, of interoperability and standardization and best practices.
Jim Frazer (17:12)
of interoperability and standardization and best practices and, you know, a lot of dry engineering approaches. So, I'm, I'm tending to think it's more evolution than a revolution at this point.
COLIN MASSON (17:32)
And I think that's pretty much the conversations we've been having internally, right? Because we look at that wide range of techniques and many of them, we as let's just say, mature engineers at ARC have experience with many of these AI tools and techniques where they weren't always called AI. But...
We now like to frame it as the industrial AI toolbox, if you like, of which some of the latest generative AI, yes, it's got use cases. And I think we should talk a little bit more about generative AI, because I think I agree with you, Jim.
If I think about industrial AI and the toolbox more broadly, it's evolutionary. The addition of the transformer technology tool set and the emergence of commercially available at scale large language models is an evolutionary step. But as I look at the impact it's having on
our daily lives on the way we interact with other technologies, even lots of our vendors who have been around for decades and used many of these other tools for decades are starting to apply Gen .ai as the new UI.
It's becoming the way that you interact with many systems. I no longer have to learn this really complex UI. I can just ask a question and it will go into...
Jim Frazer (19:08)
you're exactly right. You're -
Exactly. And that actually, actually Colin, the, you know, the next question we, we, for our audience, we only had a list of, five or six questions. we intended this to be a very conversational, approach to the topic. But, you know, one of the questions I had was in the industrial world, is there the same excitement and hype about generative AI in the industrial sector that we see?
COLIN MASSON (19:38)
the same excitement and height of that generative AI in the industrial sector.
Jim Frazer (19:46)
in the world of smartphones and chat GPT and perplexity and everything else.
COLIN MASSON (19:46)
in.
and complexity.
Well, I think by nature engineers are very measured in their approach, but what I would highlight is that...
In our survey, we do our kind of annual end of year survey, you know, typically we're getting 500, 600 to a thousand responses from industrial organizations. And we kind of ask them a fairly consistent set of questions about which technologies are going to be the most impactful, their approach to sustainability, et cetera, right? We ask them that set of questions every year. And for the last,
three years I think it is now, AI has been the most impactful technology. So long before, I think just reinforcing our point, right, that that gen AI is kind of adding, it's not revolutionary to us in the industrial sector. So I think you don't quite see the same.
that they're not quite as receptive in a good way. They're kind of more measured in, all right, what is GenAI good at and what is it not good at?
And so I think there has been excitement about the potential of AI for the last, at least the last three years as it's shown up at the top of our most impactful technologies that they're going to invest in. But we did see a big jump, almost a 35 % jump in its lead, right? It's by far the leader in terms of the most impactful technologies at the end of our 2023 survey. So there's definitely,
Jim Frazer (21:39)
Absolutely.
COLIN MASSON (21:45)
increased excitement about the potential of AI with the addition of GenAI technologies, but I think it's a lot more measured, right? Because we're engineers and we do operate mission critical and hazardous, you know, you can't just, you know, a chat bot that says the wrong thing or gives the wrong advice has a very different potential impact if you're operating a piece of equipment and hazardous chemicals, for example. So we have to
Jim Frazer (21:54)
So, yeah.
COLIN MASSON (22:15)
be a lot more rigorous in terms of evaluating it.
Jim Frazer (22:19)
So Colin, you mentioned the polls and the surveys that ARC issues each year. What does ARC research show about the adoption of industrial AI?
COLIN MASSON (22:37)
So that was a specific research project that I undertook to kind of set the stage with the industrial AI revolution, kind of in many cases just defining AI and reminding everybody that it's another tool in the AI toolbox for the industrial sector. But we did want to get some assessment of really what were the best practices.
out there in terms of what we'll let's call them leaders. I know not everyone likes to think of themselves as a leader or a laggard, right? But there are clearly those that are further ahead in their digital transformation journey and maybe have laid better foundations with connecting devices and ensuring data quality that are well poised, right, to deploy AI. So we kind of wanted to test that hypothesis and see where
the industrial sector was and so we conducted a survey of 525 industrial organizations. So we didn't look at finance sector and retail sector, it was very much focused on what we specialize in as an analyst firm. And we really zeroed in on 25 industrial AI use cases. And I think we're going to talk about those use cases maybe more in depth in a
a future podcast, but we kind of framed 25 use cases. There's a lot more than 25 use cases, but we took 25 representative use cases, which we knew from conversations with clients and commercially available solutions that they were real use cases that were being deployed today. And then we kind of wanted to see, all right, well, how widely are they being deployed? And is there a different rates of
adoption in different verticals. We also looked a little bit at, we did want to identify how far ahead are leaders because the other.
hypothesis that we went in with was really that there was a digital divide kind of has been emerging maybe over the last four or five years with we see it on digital transformation in general right and we kind of made the hypothesis that AI could widen that divide because they're so far ahead in terms of their digitalization of processes and data that they can
COLIN MASSON (25:20)
just say flip the switch but if they choose to invest in AI they have less barriers to do so and so we wanted to test that hypothesis and without going too deep yes right we found a pretty significant gap between the leaders and the laggers and there is a real indication that that digital divide if you don't act quickly the laggers are just going to well what happens
Jim Frazer (25:27)
Certainly.
COLIN MASSON (25:50)
happens, right? They don't typically disappear, but we think merger and acquisition activity could increase significantly because they're really just not going to be able to keep pace.
Jim Frazer (26:02)
So Colin, going back to the title of our initial episode, Industrial AI, Evolution or Revolution? So should we consider it Industrial AI, Evolutionary or Revolutionary?
COLIN MASSON (26:16)
Well, I think I've given you both perspectives, Jim, right? And maybe I'd ask you on your take, I tend to, I started off with thinking it's more evolutionary and the more I look at its impact and the response I'm seeing probably more from the vendors than maybe end users, right? And that's maybe something we should talk about. You know, maybe the...
The technology in some ways is ahead of the demand, right? But really, yes, there's a lot you can now do with GenAI technology. Look at how much is being invested in data centers and capacity. But then you look at the adoption, and then you've got the leaders who are really potentially widening that gap. But if you look at the main...
sector, we've really only scratched the surface of AI deployment in a lot of those use cases, you know, predictive maintenance and quality, right? So, I could play it both ways, Jim, right? It's kind of like...
Jim Frazer (27:25)
I mean, I do think, yeah.
Yeah. Yeah. so can I, the, the conservative engineer in me says, this is, a repackaging and layering on of existing technologies that have been around for decades. That's on one hand, but then I think about the, the, the application story of say the smartphone. We didn't know we needed one when it first came out.
We didn't know we needed one when it first came out. And when it came out, it was cool. It had a calculator and it had, it could make videos, different things. Well, we couldn't have imagined what it does today in your pocket. So the artist in me says, well, I probably can't imagine what AI may be able to do in the future. I can't it's, it's, you know, beyond my, my cognitive powers right now. So.
Jim Frazer (28:22)
But I trust that there will be solutions that are beyond what I can imagine. And for that, and that in fact is revolutionary. So that's, you know, my, my perspective on it. you know, thank you, Colin. This is our first episode of a, again, a five episode series. This was industrial AI evolution or revolution are episode two coming up very soon.
This is our first episode of a five episode series. This was Industrial AI Evolution for Revolution.
Coming up very soon is AI wars and industrial AI battlefronts. In episode three, we will tackle industrial AI use cases. Episode four, industrial grade data fabrics and AI. And I know Colin has an awful lot of very compelling information to share with us about that. And we will wind up this particular series with.
the industrial AI role in power and sustainability.
Jim Frazer (29:20)
And I'll contribute some of my thoughts about sustainability in that episode. So this is a five episode series and it is available both on ARC's The Sustainability Podcast as well as on the Industrial Digital Transformation Podcast. Look for it all on all of your favorite podcast players. And of course this video will also be on YouTube.
Jim Frazer (29:48)
So you can see Colin's brilliant graphic that he had on behind him for, for most of our podcast today. Thank you again for, for joining us on this and our future episodes. typically I will, I will ask our guests to share their contact information. How could somebody get ahold of you, Colin, if they, if they'd like to share more with you.
COLIN MASSON (29:58)
Typically, I will ask our guests to share their contact information. How could somebody get ahold of you, Colin, if they'd like to share more with you? Two ways. I'm very active on LinkedIn, and so you can get hold of me on LinkedIn.
My name is Colin Masson, just look for me there. The only other Colin Masson you may find, I don't think is on LinkedIn, is actually a jazz musician in the UK. So you should have no trouble finding me on LinkedIn. Or you can actually email me at cmasson .com. So that's c -m a -s -s -o -n at a -r -c -web .com.
So those are probably the two best ways to get hold of me or I'll see you at our forum or at some of the other events I get to throughout the year.
Jim Frazer (31:06) So once again, our guest was Colin Masson of ARC. He is our lead AI research analyst, and he'll be my guest for a continuing series about industrial AI topics. There's five in our current series, but I would expect far more than that as we move through the year. So thanks again, Colin, and thanks to all our listeners, and we'll see you again next time.